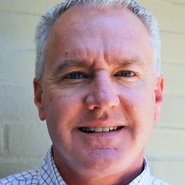
By David Dague For brands and online publishers, developing and deploying predictive modeling techniques to identify customers at-risk to churn is not rocket science. But it is pretty close. One of the simplest methods is known as “RFM” or recency, frequency and monetary. As the very terms imply, this method uses data mining to analyze and profile customers who have churned based on how recently they had made a purchase, how frequently they had purchased and their overall purchase value over time prior to defecting. These churn-risk profiles are then applied to current-customer records. Other more sophisticated methods employ multi-dimensional logistical regression and Bayesian probabilistic modeling, adding geo-demographics, psychographic panel data and feature engineering, which comprises characterizations of customer interactions with customer support, satisfaction ratings, service usage and type of products used. Still others apply statistical lifetime value forecasting and micro segmentation, and others employ almost science-fiction-sounding neural network (NN) approaches. With this level of sophistication, it is no surprise that a brand’s investment in predictive churn modeling can easily run into high-six- to seven-figure ranges and take months or more to deploy – with widely varying success rates. But there is a better, faster, cheaper way. Appetite For brands including retailers, restaurants and hospitality providers, you no longer need to predict which customers are defecting to your competition. You can know – conclusively and at the individual level which of your customers is going to competitors – each and every day. With the rapid adoption of smartphones, brands have naturally developed digital assets, namely mobile-adapted Web sites and mobile applications to meet customer needs and market products and services. It is the mobile app, however, that can deliver the deepest real-world, location-based insights that uncover where customers go and when, in a privacy compliant manner. For the first time, using app-enabled location based analytics, brands can know conclusively, which of their customers are patronizing their competitor – any competitor – when, where and how often. In short, they can know – and not guess or predict – which customers are actually leaving them to spend their money elsewhere. But that is only half of the story. Rundown As importantly, these analytics give brands insight into the interests and preferences of defecting customers based on anonymous behavioral histories of where they go and what they do in their daily lives. For example, they can know that one customer frequently participates in 10K running races, while another frequently attends wine festivals and another enjoys attending live jazz events. By combining this knowledge in addition to demographics and purchase history, brands now have the power to win-back customers and share of wallet using informed and compelling messaging and in-the-moment location-based outreach. Following the previous examples, a sporting goods retailer can tailor offers around high-end running apparel, a restaurant chain can personalize offers around wine specials and a hospitality provider can deliver e-itineraries featuring jazz clubs close to it properties. So, if you are investing thousands of dollars or more in technology and human capital to predict which customers may churn, it may be time to stop guessing, and start knowing. David Dague is vice president of marketing at Gravy, Leesburg, VA. Reach him at ddague@corp.findgravy.com.